Breaking barriers in aortic valve imaging: non-contrast CT segmentation
Challenge: accurate aortic valve imaging and reconstruction
Non-contrast CT scans are essential for patients who cannot receive contrast agents, particularly for calcium scoring in heart disease diagnosis. However, the poor visibility of the aortic valve and root in this cardiovascular imaging modality poses segmentation challenges that affect assessing conditions like aortic stenosis. Traditional manual segmentation is time-consuming and prone to errors, which limits its effectiveness.
What we did: an innovative approach to aortic valve segmentation
Graylight Imaging addressed this challenge with a semi-automated method to generate ground truth data through image registration, enabling the training of machine learning (ML) models specifically for non-contrast CT scans. By utilizing weakly supervised learning, our neural network accurately segments the aortic valve and aortic root based on non-contrast images. The quality of segmentations is assessed by employing an innovative evaluation method that aligns segmented masks from both contrast and non-contrast images.
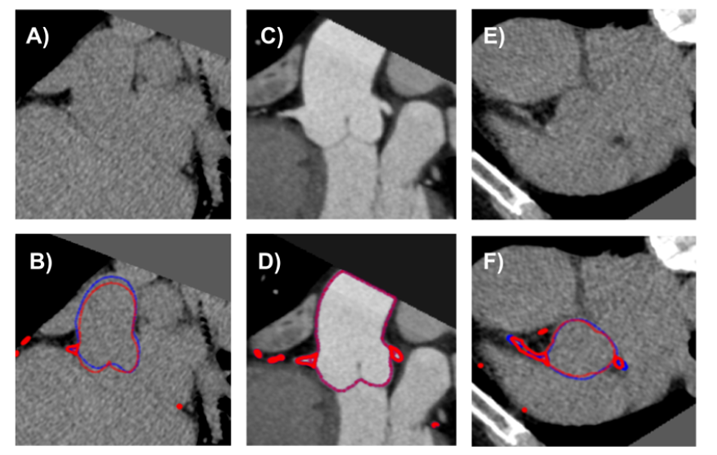
Fig. 1: Contrast-enhanced cardiac CT images. Reveal the clear delineation of the aortic root and valve (C), juxtaposed against the indistinct outlines in non-contrast images (A and E). The segmentation masks are shown in red, depicting the transformed segmentation from contrast CT (D), aligned to the non-contrast series in (B) and (F) using the Iterative Closest Point (ICP) method. The blue masks in (B) and (F) highlight the aortic root segmentation as predicted by our ML model, which operates exclusively on non-contrast CT data, demonstrating high accuracy in shape extrapolation.
Aortic root segmentation: a scalable solution
Our ML model, obtained through the scalable, semi-automatic framework, successfully segments the aortic root in non-contrast images. Achieving a mean distance error of 0.8 mm for the aortic valve. This high level of accuracy, verified through iterative closest point (ICP) alignment, demonstrates the model’s suitability for applications in coronary disease assessment and radiotherapy planning, marking a significant advancement in non-contrast medical imaging.
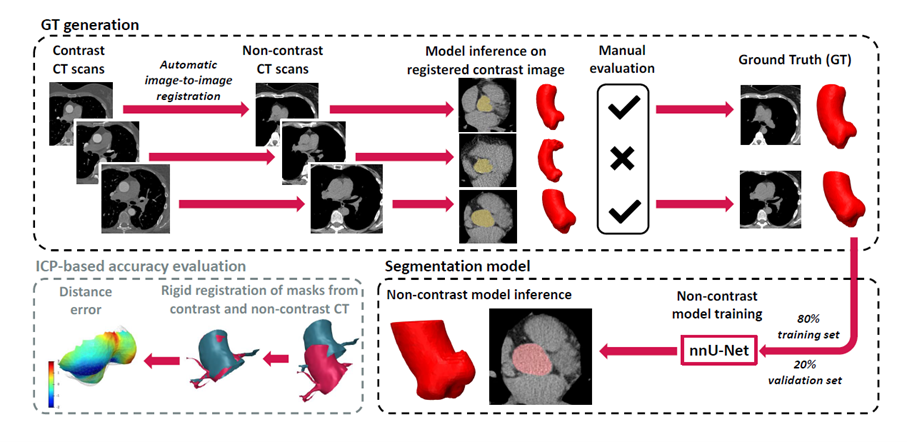
Fig.2: Semi-automatic ML model training framework and a novel evaluation technique for the aortic valve segmentation.
Optimizing scalability and efficiency
Our approach automates the processing of large datasets, requiring minimal human input. After initial registration, annotators review the results, with an average review time of just one minute per scan. This process is both efficient and highly scalable.
Data generating
By automatically segmenting aortic roots in contrast-enhanced scans, we created reliable input data for our model. Additionally, these contrast-based segmentations allow for effective registration with non-contrast images. Forming a solid foundation for training and ensuring high segmentation accuracy.
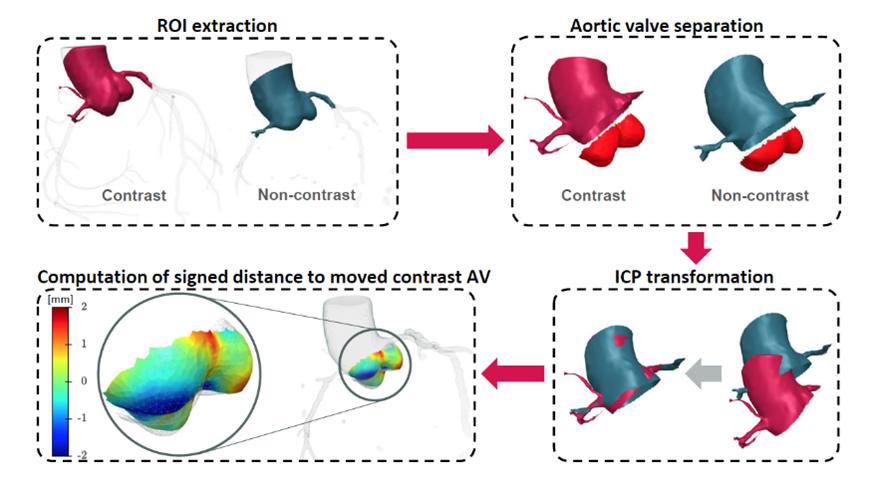
Fig.3: Evaluating the accuracy of aortic valve segmentation.
Results: a breakthrough in aortic valve segmentation
Our ML model achieved a mean distance error for the aortic valve segmentation of just 0.8 mm, making it viable for clinical use. Particularly in non-contrast CT for coronary and aortic valve calcium scoring, as well as for radiotherapy planning. This approach significantly enhances accuracy in cardiac imaging. Including aortic valve delineation, even when traditional methods fall short, establishing new standards for non-contrast imaging applications.
References:
The source of the images and all information contained in the text is the following paper: Bujny, M., Jesionek, K., Nalepa, J., Bartczak, T., Miszalski-Jamka, K., Kostur, M. (2024). Seeing the Invisible: On Aortic Valve Reconstruction in Non-contrast CT. In: Linguraru, M.G., et al. Medical Image Computing and Computer Assisted Intervention – MICCAI 2024. MICCAI 2024. Lecture Notes in Computer Science, vol 15009. Springer, Cham, https://link.springer.com/chapter/10.1007/978-3-031-72114-4_55.